As Analysts / Scientists are you utilizing fairness, ethics, accountability and transparency (FEAT)?
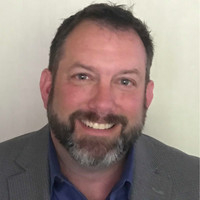
Please leave a comment if you have thoughts on FEAT affecting machine learning and concerns around the AI space within your work.
Answers
-
Assessing FEAT during the development of AI and ML applications should be required but many organizations only consider FEAT after the algorithm is finalized and use a retrospective review.
This is further complicated by academia's lack of focus on data science ethics (Macaulay, 2020).
Assessing FEAT during each step of the data science process (be it CrISP-DM or KDD or something else) should be ingrained into every data science student and all practicing data scientists.
References:
Macaulay, T. (2020). Study: Only 18% of data science students are learning about AI ethics. The Next Web. https://thenextweb-com.cdn.ampproject.org/c/s/thenextweb.com/neural/2020/07/03/study-only-18-of-data-scientists-are-learning-about-ai-ethics/amp/
3 -
Basically agree with @Michael McCarthy I would add that xAI or explainable AI is an important part of this. One needs to be able to understand and explain how the AI is working, which includes the data that was used to train it. Google xAI for many excellent discussions of this. The one I most often refer to is this one from DARPA. https://www.darpa.mil/program/explainable-artificial-intelligence
3